Criminal Risk Assessments: Secret Algorithms, Flawed Results and a Black-Box Future
BY Ryan Conley
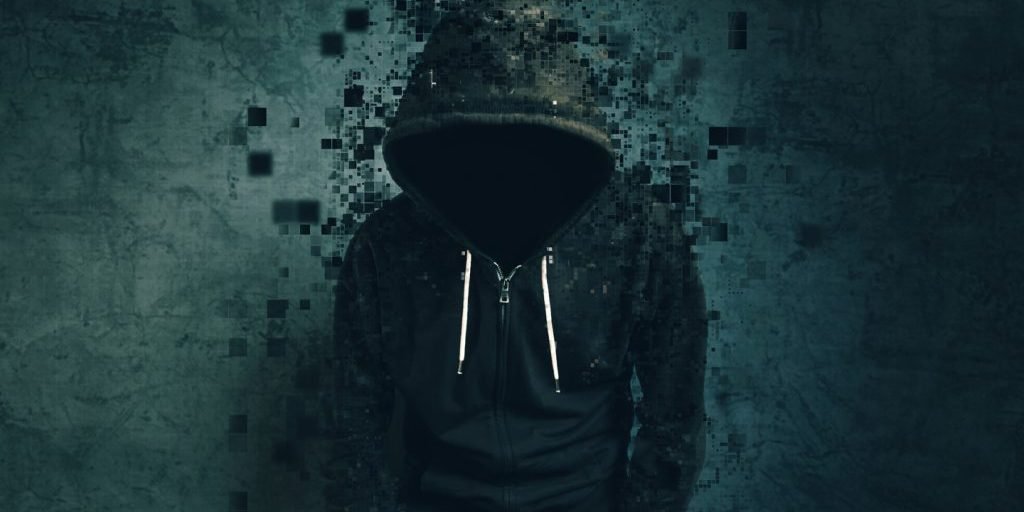
LISTEN
Increasingly, criminal sentencing decisions are guided in part by sophisticated computer algorithms that aim to predict, among other things, a convict’s likelihood to re-offend. While the likelihood of recidivism is clearly central to sentencing, some are sounding alarms over the opacity of the so-called “risk assessments,” which are often derived from formulas that are proprietary and secret.
Do algorithms violate due process?
Eric Loomis received a six-year prison term following a 2013 arrest for fleeing from the police in a stolen car in La Crosse, Wisconsin. The judge said that Loomis presented a “high risk” to the community and based his sentence in part on his COMPAS score. COMPAS is an algorithm developed by Northpointe, a for-profit company. The algorithm is aimed at predicting recidivism based on the answers to 137 questions in categories ranging from “criminal history” to “education” and “residence/stability.” The exact way in which these answers are factored into a defendant’s “risk score” is a trade secret.
Loomis argues that because he is unable to review the algorithm and challenge its scientific validity, its use in his sentencing is a violation of his right to due process.
He also contends lack of due process in that COMPAS includes gender as a factor in predicting recidivism, as Northpointe admits.
The Wisconsin attorney general’s office defends the use of COMPAS, characterizing it as providing an assessment of risk “individualized to each defendant.” The Supreme Court of Wisconsin in 2016 upheld this argument, ruling against Loomis on the basis that the assessment provided useful information and was just one component of his sentencing decision.
Loomis has a petition pending before the U.S. Supreme Court, which has shown interest in the case. In March, the Court invited the Acting Solicitor General to submit a brief expressing the views of the United States. That brief, filed in May, recommended the Court deny review. Although the government concedes that the risk assessments raise “novel constitutional questions,” the brief argues that Loomis would have received the same sentence even without COMPAS, and points out that only the Supreme Court of Indiana has ruled on the use of algorithms in sentencing, similarly to Wisconsin.
Public concerns, secret methodology
The purpose behind data-driven, algorithmic risk assessment in criminal sentencing is to keep people out of prison. Virginia, which has one of the longest-running algorithm risk assessment programs in the nation, began scoring defendants in 2002 in the midst of a budget crunch. Faced with overcrowded prisons and no funds to build new ones, the legislature directed the sentencing commission to develop a method for identifying offenders who were unlikely to re-offend. These low-risk offenders were then diverted away from prison into alternative sanctions including probation and house arrest.
The sentencing commission developed a formula that when tested against historical data correctly predicted a convict’s chance of re-offending three out of four times, and after its implementation, Virginia’s prison population began to level off. But when some offenders are deemed less likely to re-offend, it follows that others are deemed more likely, and therefore more deserving of a lengthy prison sentence. It is this concern that has experts alarmed at the implications of this practice.
Today, the use of these algorithms continues to grow. Many states, like Virginia, use formulas developed by sentencing commissions or academic experts which are available for examination by the public. But other states, including Wisconsin and Florida, use algorithms which are developed by private companies and protected as trade secrets.
Although courts appear willing to uphold this protection, this does not consign these algorithms to utter secrecy. The concept of “qualified transparency” advocated by, among others, Frank Pasquale, professor of law at the University of Maryland, may provide a middle ground. Expert investigators could be permitted to examine a secret algorithm away from public view and render an opinion to the court as to its scientific soundness and constitutionality.
Questionable results
Even if we hesitate to trust computer algorithms with criminal justice, we might still give technology the benefit of the doubt. Given a sufficiently large data set, should an algorithm not be able to draw accurate correlations between a defendant’s circumstances and the many similar cases that came before? This, after all, is one of the core promises of computer decision-making: computers lack the capacity for bias, social or otherwise, and should theoretically be capable of the blindness we ascribe to Justice.
In reality, this may be far from the truth. Journalists at ProPublica conducted an investigation into risk assessment scores created by Northpointe. They obtained the algorithm-calculated risk scores of over 7,000 people arrested in Broward County, Florida and checked to see which of those people were charged with new crimes during the following two years. This is the same benchmark used by Northpointe. If the company’s algorithm works as intended, it should predict recidivism with a high degree of accuracy.
ProPublica found it did not. Of those deemed likely to re-offend, just 61 percent were arrested for any crime in the two years subsequent to their initial arrest, including misdemeanors. In other words, the algorithm was a bit more accurate than a coin toss. When it came to predicting violent crimes, the algorithm fared even worse. Just 20 percent of those deemed likely to commit violent crimes were arrested for such a crime.
Even more troubling than the algorithm’s apparent inability to predict recidivism is the disparity of the risk scores assigned to offenders of different races. Although race is not one of the data points used by Northpointe’s algorithm, ProPublica found a startling racial bias in the scores. The formula wrongly predicted black defendants would re-offend at twice the rate it did for whites. And in cases where the algorithm failed to predict recidivism that actually occurred, it did so more often with white defendants than with blacks. Northpointe disputes the analysis.
Troubling future
If sentencing decisions guided by secret algorithms is not bad enough, artificial intelligence, aka “AI” or “machine learning,” could eventually add a whole other level of opacity to the process. Even if private risk assessment companies’ algorithms remain secret, we can at least take stock in the fact that their workings are not mysterious to the company itself. The same can not be said for artificial intelligence.
Properly understood, most computer algorithms, though they can be extremely complex and difficult for any one person to understand completely, do not constitute artificial intelligence. AI works by programming a computer to alter its own programming, which can yield results that are unpredictable and sometimes even difficult to accurately describe. Moreover, given the assumption that increased accuracy is desirable, a sentencing AI might remain free to alter itself continuously. Such a system might become impossible to fully understand, even by its creators.
And what if that system, even if only in experiments, could be clearly demonstrated to predict recidivism with very high accuracy? What happens when algorithms really can predict crime? Would the use of such a system not find strong advocates among tough-on-crime politicians and prosecutors, among the public, and perhaps even among judges?
In the short term, with Loomis’ petition pending before the Supreme Court, algorithmic risk assessment and sentencing may be stymied by the more practical concern of due process. In the not-too-distant future, however, we might be forced to decide how much authority to cede to computers with predictive powers that are as remarkable as they are mysterious.
LATEST STORIES